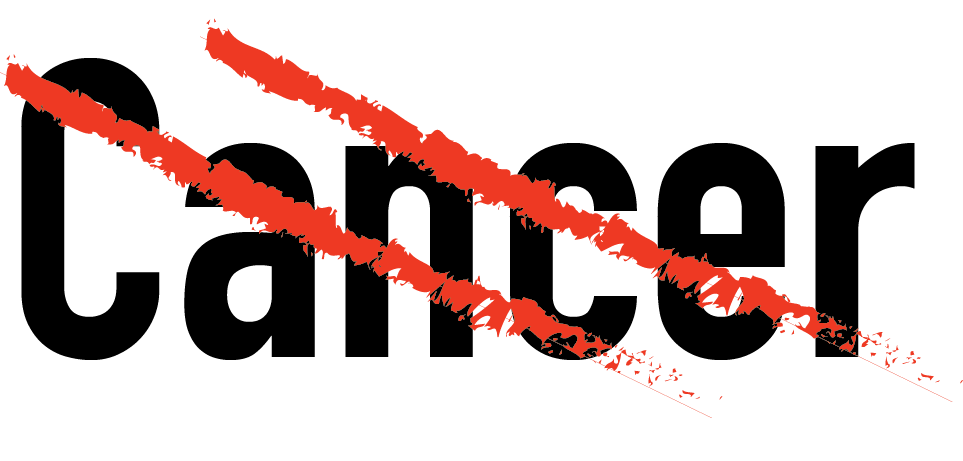
Research Interests
The research topic of my lab is on characterising mutational landscape of human gastrointestinal cancer, elucidating the association between mutational footprints and environmental factors, identifying biomarkers with translational significance in immunotherapy and early cancer diagnosis, and interpreting medical imaging data using deep learning algorithms.
Web Applications
- DECIDIA: Deep-learning for Early Cancer Interception and DIAgnosis.
- TORCH: Tumor origin determination for cellular histology.
- WIT-Attention-Maps: The attention maps of WIT on whole-slide images.
- WIT-Classifier: WIT classifier for the detection of 32 TCGA cancer types.
Github repos
- Miscell: Miscell: An efficient self-supervised learning approach for dissecting single-cell transcriptome.
- iSEEEK: A universal approach for integrating super large-scale single-cell transcriptomes by exploring gene rankings.
- Fugue: Scalable batch-correction approach for integrating large-scale single-cell transcriptomes.
- HTNet: Deep learning to diagnose Hashimoto’s thyroiditis from sonographic images.
- CRCNet: Diagnostic evaluation of a deep learning model for optical diagnosis of colorectal cancer.
Presentations
- PhD oral defence: The oral defence PPT for my PhD study in CUHK.